

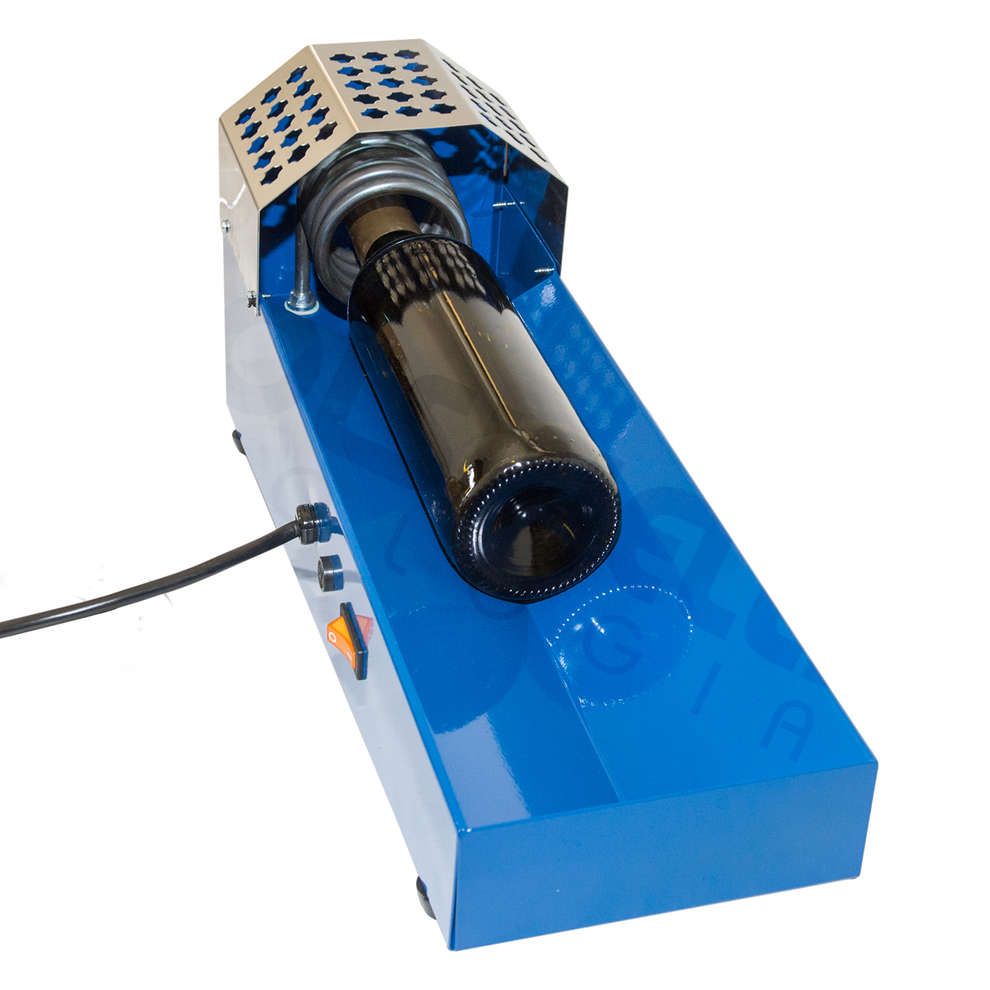
1, object translations in the input space do not affect activations of high-level neurons, because max-pooling layers are able to rout low-level features between the layers. Local shared connectivity coupled with spatial reduction layers, such as max-pooling, extract local translation-invariant features. Indeed, using translated replicas of learned feature detectors, features learned at one spatial location are available at other locations. In contrast to other deep neural architectures, the main characteristic of a CNN is its capability to efficiently replicate the same knowledge at all locations in the spatial dimension of an input image. In the last decade, convolutional neural networks (CNN) drastically changed artificial visual perception, achieving remarkable results in all core fields of computer vision, from image classification 1, 2, 3 to object detection 4, 5, 6 and instance segmentation 7. Extensive experimentation with other capsule implementations has proved the effectiveness of our methodology and the capability of capsule networks to efficiently embed visual representations more prone to generalization. Moreover, we replace dynamic routing with a novel non-iterative, highly parallelizable routing algorithm that can easily cope with a reduced number of capsules. In this paper, we investigate the efficiency of capsule networks and, pushing their capacity to the limits with an extreme architecture with barely 160 K parameters, we prove that the proposed architecture is still able to achieve state-of-the-art results on three different datasets with only 2% of the original CapsNet parameters.

Nevertheless, little attention has been given to this relevant aspect. Indeed, a properly working capsule network should theoretically achieve higher results with a considerably lower number of parameters count due to intrinsic capability to generalize to novel viewpoints. Even though capsules networks are still in their infancy, they constitute a promising solution to extend current convolutional networks and endow artificial visual perception with a process to encode more efficiently all feature affine transformations. That is highly inefficient and for large datasets implies a massive redundancy of features detectors. Deep convolutional neural networks, assisted by architectural design strategies, make extensive use of data augmentation techniques and layers with a high number of feature maps to embed object transformations.
